The Difference Between AI and Generative AI
- AI, Generative AI
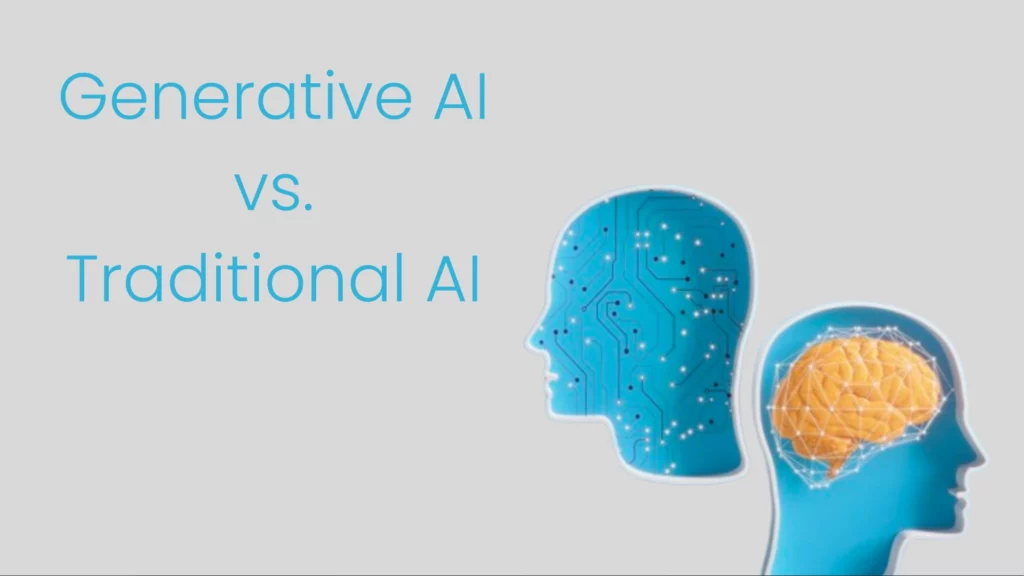
The terms Generative AI (GenAI) and Artificial Intelligence, while related, represent distinct applications within the AI landscape.
At its core, AI encompasses a broad range of technologies and techniques that enable machines to simulate human intelligence. It is the ability of a machine to learn, adapt, and make decisions based on data and information. AI addresses a wide spectrum of use cases, from self-driving cars to facial recognition software. All of these applications are powered by algorithms that enable machines to perform tasks that were once considered the exclusive domain of human intelligence.
Prefer to listen to the content?
Hear ELASTECH’s CEO, Armen Tatevosian, and EVP of Global Operations, Jim Zordani, providing clarity on the distinctions between AI and Generative AI (GenAI), shedding light on their capabilities and applications.
Generative AI, on the other hand, is a subset of AI that focuses on creating new content. GenAI systems produce original text, images, music, and even code, mimicking the creative process that humans have historically been responsible for. As a result, GenAI is often referred to as the “creative” side of AI, delivering the ability to generate ideas, concepts, and expressions, pushing the boundaries of what was previously thought possible by incorporating broad data sets into new perspectives.
The primary distinction between AI and GenAI lies in their outputs. While the former focuses on making decisions, predictions, or classifications based on existing data, Generative AI takes a different approach to predicting and prescribing entirely new content.
How Does Generative AI Work?
GenAI systems typically rely on a technique called deep learning, which involves training systems called artificial neural networks using massive amounts of data, often referred to as Large Language Models. These neural networks recognize patterns and relationships within data to generate new content that is similar to the training data but with a degree of originality. Essentially, GenAI is learning the underlying structure and patterns of existing data to use that knowledge and create new, original works.
Large Language Models
The term Large Language Models (LLMs) can often be heard in association with Generative AI. LLMs are massive neural networks trained on vast amounts of publicly-accessible, textual data. They generate text, translate languages, write different kinds of creative content, and answer your questions in an informative way. These characteristics are being used in GenAI systems like Bard, Claude, and ChatGPT, where LLMs provide the underlying intelligence to create new text content.
Use Cases of AI and Generative AI
There is a wide range of applications making use of Generative AI, from generating personalized marketing materials to developing new forms of art and entertainment to writing entire code bases. GenAI is also increasingly being leveraged in customer support. Learn more about how and where Generative AI delivers real business value.
While the use cases of GenAI are diverse, they are limited to the development of content. This is a key difference from “traditional AI,” as applications cover a wider range of use cases and business needs. There is an increasing adoption of AI in fraud detection, KYC, and process automation, as well as document audit and processing.
In contrast to “traditional AI,” the barrier to entry is non-existent for the use of Generative AI, with a publicly-available user interface in applications like ChatGPT, Google Bard, and Claude.ai. Each of these GenAI applications utilizes a different Large Language Model: Claude.ai employs Claude, Google Bard was built on BERT, and ChatGPT utilizes GPT-4. The primary differentiator among these popular tools lies in the training methodologies used in their respective LLMs.
It is important to note that GPT-4 is not exclusively accessible through ChatGPT. Companies have begun developing applications that interface with this LLM via APIs, enabling them to leverage the data for their own purposes, such as generating on-brand marketing copy without making proprietary data available. This highlights the potential for companies to leverage pre-existing Generative AI models to their advantage.
The Functionality of Popular Generative AI Tools
A critical component of popular GenAI applications, like ChatGPT, is Natural Language Processing (NLP), a branch of Artificial Intelligence dedicated to comprehending and generating human language. NLP techniques enable GenAI systems to process and analyze contextual data, extracting meaning and identifying patterns. This understanding of language is essential for generating new text that is both coherent and relevant within a context.
ChatGPT, for instance, utilizes a combination of NLP techniques, including:
- Tokenization: NLP breaks down text into individual units called tokens, which can be words, punctuation, or even individual characters.
- Part-of-speech tagging: This process refers to labeling each token with its grammatical role, such as noun, verb, adjective, or adverb.
- Dependency parsing: Natural Language Processing identifies the relationships between words in a sentence, creating a structured representation of the sentence’s meaning.
- Named entity recognition: This NLP technique recognizes and classifies named entities within text, such as people, places, or organizations.
In addition, ChatGPT employs a technique called “probabilistic language modeling,” which assigns probabilities to the next word in a sequence. This allows the model to generate text that is not only grammatically correct but also stylistically consistent with the input.
Conclusion
AI is a broad term that encompasses a range of technologies and techniques to simulate human intelligence. GenAI is a subset of AI that focuses on creating new content. The primary distinction between AI and GenAI lies in their output: AI makes decisions, predictions, or classifications based on existing data, while GenAI creates new and unique content. As a result, the applications of “traditional” AI are wider-ranging than those of GenAI systems. Generative AI applications, such as ChatGPT, Claude, and Bard, are built on Large Language Models (LLMs), which are massive neural networks trained on vast amounts of publicly accessible data. These popular GenAI tools use NLP techniques to process and analyze contextual data, extract meaning, and identify patterns. This understanding of language is critical for generating new content that is both coherent and relevant within a given context.
We often hear from leaders that, while they have heard a lot about AI, they are concerned that they do not have an adequate understanding of what AI can really do for them in their business context. ELASTECH is a company recognized for delivering game-changing AI solutions and for its commitment to fostering a deeper understanding of AI.
We invite you to take a deeper dive into the practical applications for your organization. Our experts will help you evaluate and confirm specific opportunities with AI to deliver value to you. Click below to schedule a free AI consultation.
Schedule your Free Consultation with an AI Expert